I graduated with a Master’s degree in Artificial Intelligence from Northwestern University in December 2024. My primary research area is computer vision, with a keen interest in multimodal research. I focus on developing robust and generalizable representations for computer vision and adjacent modalities, including but not limited to self-supervised learning, representation learning, unsupervised learning, and meta-learning.
In the summer of 2024, I worked as a visiting graduate student at Johns Hopkins University under Prof. Alan Yuille at the Computational Cognition, Vision, and Learning (CCVL) lab, working on hyperbolic deep learning in computer vision. At Northwestern, I am a Research Assistant under Prof. Lee A. Cooper at the Computational and Integrative Pathology Group, focusing on panoptic segmentation of histopathological images.
I completed my Bachelor of Technology in Mechatronics Engineering with a minor in Computing from Manipal Institute of Technology, India. During my bachelor’s, I worked with Prof. Biplab Banerjee at the Indian Institute of Technology - Bombay on meta learning for super resolution and domain adaptive few shot learning. I was also an active member of AeroMIT, a multidisciplinary aerial robotics team at Manipal Institute of Technology, where I led the Autonomous Drone Research subsystem.
Currently, I am actively working on Multi-Agent Systems at CAMEL-AI. In my free time, I write for The Batch by deeplearning.ai.
If you’d like to chat, please feel free to reach out at deeptej[at]u.northwestern.edu
Recent Publications
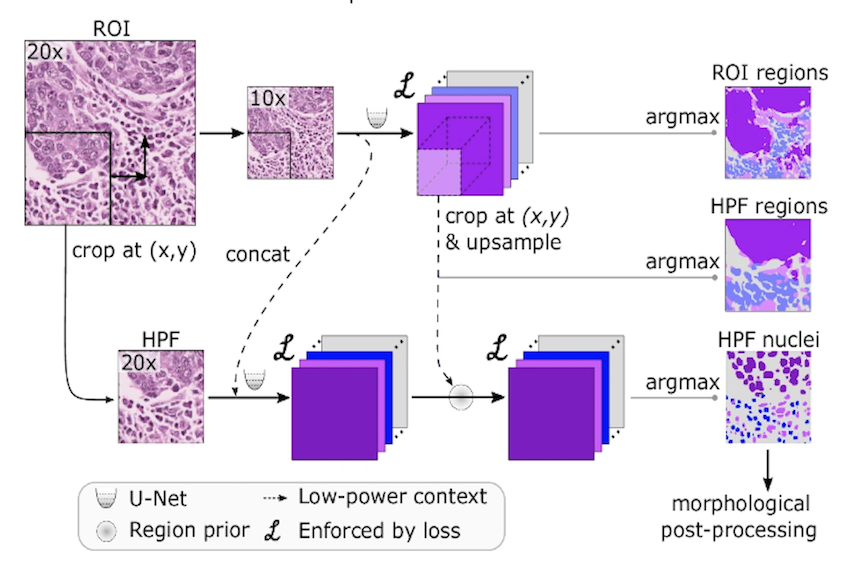
A panoptic segmentation dataset and deep-learning approach for explainable scoring of tumor-infiltrating lymphocytes
npj Breast Cancer, June 2024
A deep-learning approach for panoptic segmentation and scoring of tumor-infiltrating lymphocytes in breast cancer.
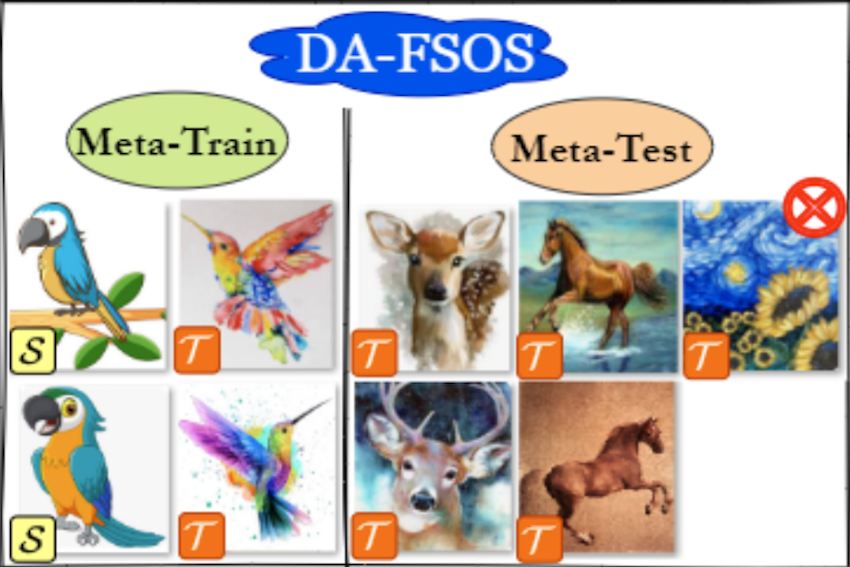
Domain Adaptive Few-Shot Open-Set Learning
ICCV 2023, October 2023
A Meta-Learning based framework powered by Generative Adversarial Networks for recognizing unknown samples from novel classes in target query sets.
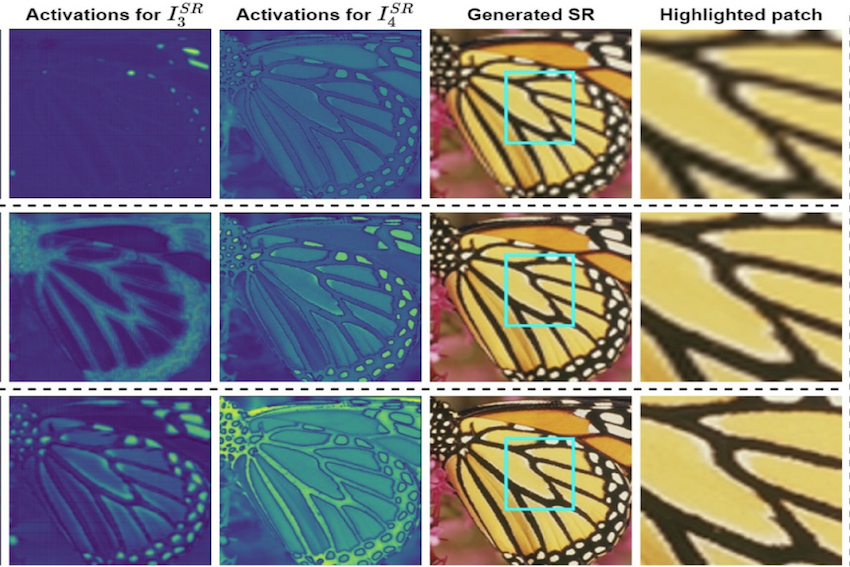
MAML-SR: Self-adaptive super-resolution networks via multi-scale optimized attention-aware meta-learning
Pattern Recognition Letters, September 2023
A Meta-Learning based multi-scale framework to solve the problem of insufficient adaptability of deep-learning-based super-resolution methods to novel blur kernel scenarios.